일 | 월 | 화 | 수 | 목 | 금 | 토 |
---|---|---|---|---|---|---|
1 | 2 | 3 | 4 | 5 | ||
6 | 7 | 8 | 9 | 10 | 11 | 12 |
13 | 14 | 15 | 16 | 17 | 18 | 19 |
20 | 21 | 22 | 23 | 24 | 25 | 26 |
27 | 28 | 29 | 30 |
- q learning
- 카멜케이스
- 배반사건
- 헝가리안노테이션
- 도커 컨테이너
- 강화학습
- 몬테카를로 학습
- mmoe
- 스네이크케이스
- 코딩스타일
- Traveling salesman problem
- 유전알고리즘
- 케밥케이스
- 연합학습
- 그리드월드
- 파스칼케이스
- 딥러닝
- routing problem
- Docker Image
- 확률공리
- Sarsa
- Metaheuristic
- on-policy
- multi task learning
- 산업공학
- 큐러닝
- 도커 개념
- genetic algorithm
- Federated learning
- off-policy
- Today
- Total
SU Library
[연합학습] Federated Learning의 개념과 간단예제 본문
이런저런 일로 매우 한동안 너무 바빴어서 이제서야 포스팅을 해봅니다. 오늘은 Federated Learning (FL)이라는 개념에 대해 알아보고자합니다. 연합 학습(Federated Learning)은 각데이터를 공유하지 않고 탈중앙화 장치 또는 데이터 소스에서 머신 러닝 모델을 훈련하는 데 사용됩니다.
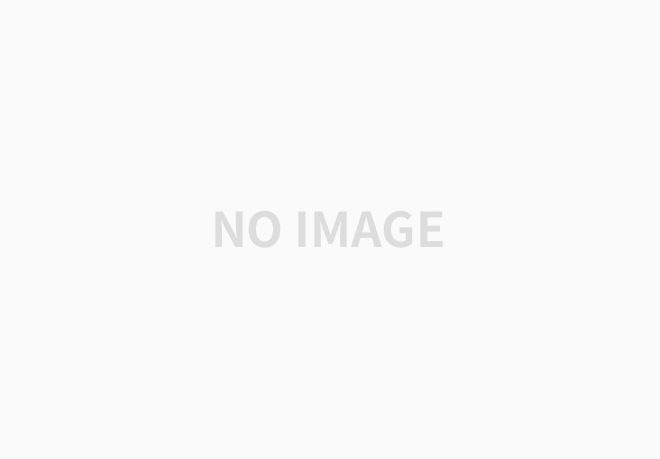
즉, 여러대의 디바이스들이 존재한다고 가정할때, 이들의 데이터가아닌 이들이 가진 가중치들을 서로 공유함으로써 글로벌 모델은 거시적인 인사이트를 얻고 이를 모든 디바이스들에게 공유하는 방식인 것입니다.
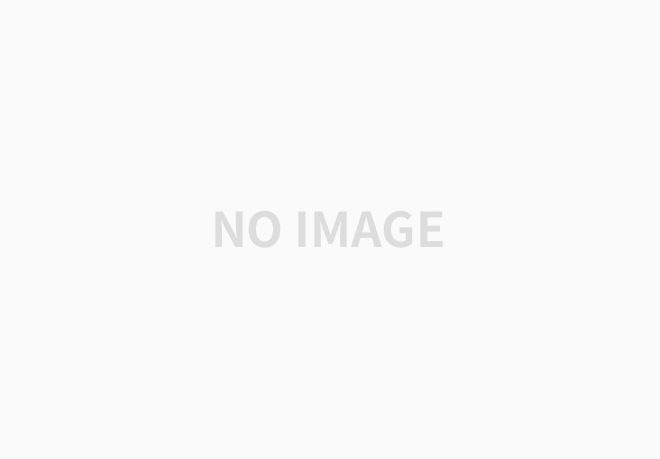
이는 다음과같은 이점들이 있습니다.
개인정보 보호 및 보안: 데이터를 로컬로써 유지하고, 사용자 개인정보와 같은 민감한 정보들에 대한 보호를 보증합니다.
탈중앙화: 데이터를 중앙 집중화하지 않고도 여러 장치에서 학습한 가중치를 공유할 수 있습니다.
효율성: 모델 업데이트만 공유하여 대역폭과 리소스를 절약하여 데이터 전송을 줄입니다.
개인화: 글로벌 모델에 기여하면서 로컬 데이터에 맞는 모델을 만듭니다.
확장성: 수천 개의 클라이언트로 확장되며 IoT, 엣지 디바이스 및 대규모 네트워크에 이상적입니다.
협업: 민감한 데이터를 공유하지 않고도 함께 모델을 학습할 수 있습니다.
FL은 의료, 모바일 앱, 금융 및 IoT에 사용되며 개인 정보 보호, 효율적이고 협업적인 머신 러닝 솔루션을 제공합니다.
이제 간단 실습 예제 코드를 살펴보겠습니다.
import tensorflow as tf
from tensorflow.keras import layers, models
import numpy as np
import warnings
warnings.filterwarnings('ignore')
# Load CIFAR-10 dataset
(x_train, y_train), (x_test, y_test) = tf.keras.datasets.cifar10.load_data()
# Normalize images to the range [0, 1]
x_train = x_train.astype('float32') / 255.0
x_test = x_test.astype('float32') / 255.0
# One-hot encode labels
y_train = tf.keras.utils.to_categorical(y_train, 10)
y_test = tf.keras.utils.to_categorical(y_test, 10)
print(f"Train shape: {x_train.shape}, {y_train.shape}")
print(f"Test shape: {x_test.shape}, {y_test.shape}")
cifar-10데이터셋을 Import 합니다.
def split_data(x, y, num_clients):
client_data = []
shard_size = len(x) // num_clients
for i in range(num_clients):
start = i * shard_size
end = start + shard_size
client_data.append((x[start:end], y[start:end]))
return client_data
# Split data for 3 clients
num_clients = 3
client_data = split_data(x_train, y_train, num_clients)
연합학습을 구현하기위해 3개의 디바이스들을 사용한다고 가정하고 임의로 학습 데이터를 3개로 분할합니다.
def create_model():
model = models.Sequential([
layers.Conv2D(32, (3, 3), activation='relu', input_shape=(32, 32, 3)),
layers.MaxPooling2D((2, 2)),
layers.Conv2D(64, (3, 3), activation='relu'),
layers.MaxPooling2D((2, 2)),
layers.Flatten(),
layers.Dense(64, activation='relu'),
layers.Dense(10, activation='softmax')
])
model.compile(optimizer='adam', loss='categorical_crossentropy', metrics=['accuracy'])
return model
평범한 CNN모델을 정의합니다.
# Number of rounds for federated learning
num_rounds = 10
for round_num in range(num_rounds):
print(f"Round {round_num + 1}/{num_rounds}")
local_weights = []
# Train on each client
for client_id in range(num_clients):
print(f"Training on client {client_id + 1}")
# Create local model and set global weights
local_model = create_model()
local_model.set_weights(global_model.get_weights())
# Get client data
X, y = client_data[client_id]
# Train local model
local_model.fit(X, y, epochs=1, batch_size=32, verbose=0)
# Collect local model weights
local_weights.append(local_model.get_weights())
# Federated averaging: Aggregate local weights
averaged_weights = [np.mean([local_weights[j][i] for j in range(num_clients)], axis=0)
for i in range(len(local_weights[0]))]
# Update global model weights
global_model.set_weights(averaged_weights)
# Evaluate the global model
loss, accuracy = global_model.evaluate(x_test, y_test, verbose=0)
print(f"Global model accuracy after round {round_num + 1}: {accuracy:.4f}")
3개의 디바이스를 사용하여 10번 반복하며 학습을 진행합니다. 수집된 로컬 가중치들은 평균화하여 global 모델에 업데이트되고, global모델의 가중치를 다시 디바이스들에게 배포하는 식으로 학습을 진행합니다.
Round 1/10
Training on client 1
WARNING: All log messages before absl::InitializeLog() is called are written to STDERR
I0000 00:00:1733186640.922935 71 service.cc:145] XLA service 0x798e20005fc0 initialized for platform CUDA (this does not guarantee that XLA will be used). Devices:
I0000 00:00:1733186640.922991 71 service.cc:153] StreamExecutor device (0): Tesla P100-PCIE-16GB, Compute Capability 6.0
I0000 00:00:1733186642.990420 71 device_compiler.h:188] Compiled cluster using XLA! This line is logged at most once for the lifetime of the process.
Training on client 2
Training on client 3
Global model accuracy after round 1: 0.4377
Round 2/10
Training on client 1
Training on client 2
Training on client 3
Global model accuracy after round 2: 0.5691
Round 3/10
Training on client 1
Training on client 2
Training on client 3
Global model accuracy after round 3: 0.6213
Round 4/10
Training on client 1
Training on client 2
Training on client 3
Global model accuracy after round 4: 0.6499
Round 5/10
Training on client 1
Training on client 2
Training on client 3
Global model accuracy after round 5: 0.6646
Round 6/10
Training on client 1
Training on client 2
Training on client 3
Global model accuracy after round 6: 0.6702
Round 7/10
Training on client 1
Training on client 2
Training on client 3
Global model accuracy after round 7: 0.6838
Round 8/10
Training on client 1
Training on client 2
Training on client 3
Global model accuracy after round 8: 0.6930
Round 9/10
Training on client 1
Training on client 2
Training on client 3
Global model accuracy after round 9: 0.6981
Round 10/10
Training on client 1
Training on client 2
Training on client 3
Global model accuracy after round 10: 0.7022
'인공지능 > Deep learning' 카테고리의 다른 글
[멀티테스크 러닝]Multi task learning with Multi-Gate Mixture-of-Experts 모델 개념 정리 및 구현 (0) | 2024.06.18 |
---|